Emotion is key to designing digital experiences
As a CX / UX agency, we have been working hard over the last 5 years to get deeper into the hearts and minds of users.
We've been trying to define how the next generation of digital experiences should work.
That means going beyond shiny features and whizzy functions that grab short term attention - and getting to the emotions and behaviours that really drive engagement and reduce promiscuity and dropout.
Brands that harness the power of emotion engage their customers more effectively, and branding has long been about connecting the intangible aspects of an organisation's culture and values with its audiences and customers.
But with digital adoption, many businesses seemed to forget this: they traded the power of brand for the wow of technology.
This might have created some amazing digital interaction, but often the experiences were lacked depth, durability and competitive differentiation.
An example of a brand that has failed to transfer its rich offline heritage of emotional value into its digital experiences is John Lewis.
The brand has long set the standard for exceptional store-based customer experience, but have struggled to inject the ‘humanness’ of their staff into a digital environment.
In trying to compete with convenience of Amazon and disruptors like Appliances Online (AO.com) they have created a shopping site that lacks distinctive magic, enjoyment and John Lewisness.
Other brands have done better.
Red Bull is just one great example as their online presentation of events, sponsorship and content matches the stimulating approach in its offline channels.
The outcome is a brand that connects its product with emotional values and aspirations and amplifies its message far beyond the traditional restrictions of retail marketing.
So for us, as experience designers, we need to understand how a brand can deliver more emotional impact.
This starts with understanding what turns people on and off; what makes them enthusiastic or anxious; and what drives real value beyond the basics of great prices and fast delivery.
By mapping the range of the emotional needs, we can align the brand's story with underlying customer needs and create immersive journeys that use digital channels to best effect.
Put simply, emotion is core to our projects because it gives us the ability to design experiences that matter.
So we have been trialling a range of emotion AI systems to integrate with our mix of in-person and digital research methods.
We are currently using tools to develop a fuller picture of emotional responses across customer journeys, channels, touchpoints and devices.
A few examples include analysing physical reactions such as galvanic skin response, facial expression and brain activity; deconstructing how people use different writing formats; and assessing responses to various content structures.
Some EmotionAI platforms we have used
Hotjar is very good at tracking the range of user interactions within a website, but it also provides a valuable tool for highlighting where certain emotions maybe being triggered or enhanced.
This insight can either inform hypotheses and assumptions for testing, or can be used to contextualise other research. For example, interviewees may suggest they feel very anxious early on in their experience; using the Hotjar screen recordings we might confirm a large volume of people struggle to navigate within the primary landing point.
Albert
We have been investigating a number of ways to use AI and Machine Learning for optimising PPC ad copy. Tools such as Albert offer big advantages in the speed and volume of ideas that can be tested (at a cost - this tool is not a budget friendly choice).
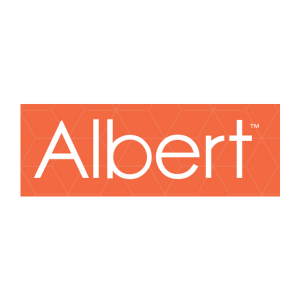
Albert gives us the opportunity to test different response types for variants of messaging, imagery and calls to action with different target audiences. It also allows us to look at which days of the week and which timeslots work best. The output gives us the optimum combination of copy, imagery, channels and timing to maximise response.
One recent experiment was for a gut health campaign targeting 30 - 35yr old women. The result showed that mums tend to be more active around noon and early evening and respond well to terms like tummy; whereas affluent professional women of the same age respond better to more assertive, scientific language such as gut health and stomach. The campaign could then be tailored and targeted accordingly.
IBM Watson
There is a growing number of text analysis tools on the scene, but we have worked mainly with IBM Watson as it is now a comparatively mature technology.
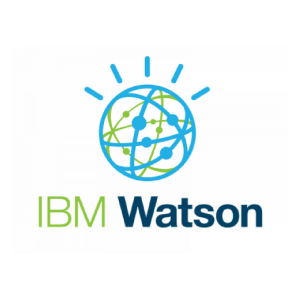
Text diagnostic analysis is a valuable component in our emotional research toolkit. It offers us the ability to cross reference assumptions from other research sources and to add new perspectives.
It helps, for example, to understand how social media impacts people's emotional state at certain points in their decision path. We are still very much in the test-and-learn phase with this type of approach, but we experiment regularly with low cost pilots on different client briefs.
Challenges and limitations in these new tools
While the opportunities these new tools bring are great, it's important to recognise some of the challenges they bring as well, namely:
- Costs in subscribing to / using some of them - it can be challenging to get buy-in from clients to fund longer term evaluation
- Lack of consistent taxonomy for emotional response types
- Difficulty in reconciling results across different tools
- Building a consolidated view from multiple data sources
- Complexity of training and ownership as insights can pull from a wide range of departments in an organisation
- Working out which systems produce quality results and which just create superficial outputs
- Applying and maintaining systems long enough to fully establish patterns
- The personal privacy dimension is only going to more sensitive: how much monitoring and analysis is appropriate; how much is personally identifiable; and do you have enough informed consent in place?